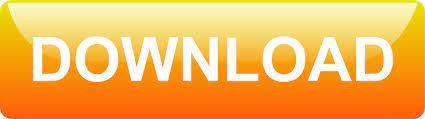
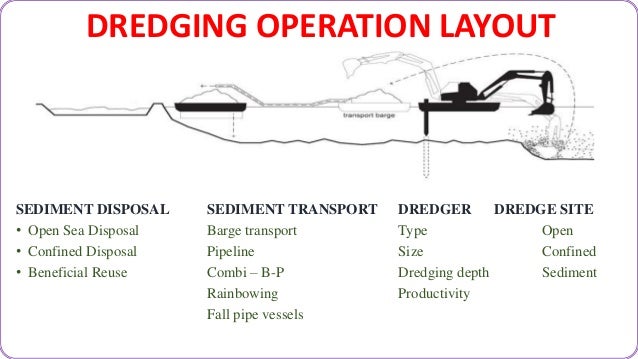
This practice is to some extent unavoidable, as in our structural break example. In applications, a possible distribution for the data is usually not chosen ex ante, but ex post by eye-balling a histogram. They illustrate the practice with Pearson’s \(\chi ^2\) goodness-of-fit (GoF) test.
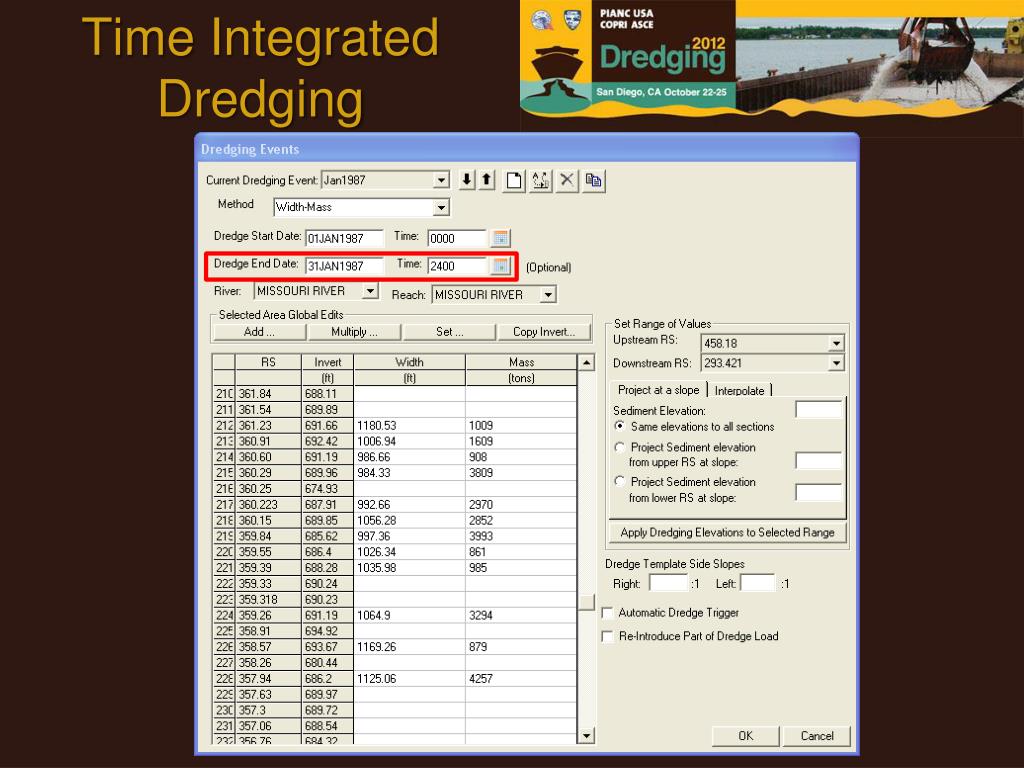
Selvin and Stuart ( 1966) call this ‘hunting’, because the investigator hunts for hypotheses to be tested based on the data. We mention that the issue of investigating the data to ‘decide’ which hypotheses to test is an old one.
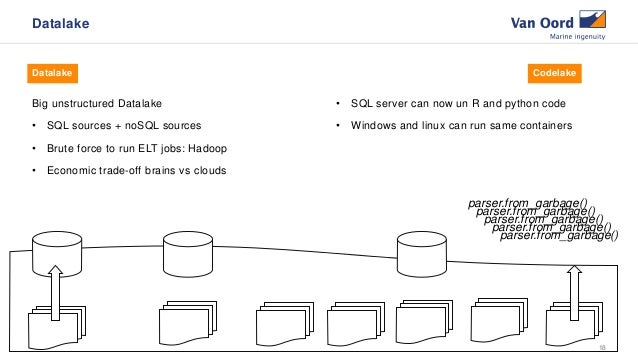
The second main aim of this paper is to show that, unlike for one-shot structural break tests, this is indeed the case. Then, similarly as above, the monitoring procedure needs to hold size conditionally on large fluctuations in the training data. One possibility may be to monitor a parameter, whose estimates have fluctuated somewhat in the training data. Of course, ex ante it may be unclear precisely which parameters to monitor for constancy. This changes when one moves from a structural break context, where all data are available in advance, to a monitoring context, where-after having observed some training data-the data become available ‘as you go’ for sequential tests of parameter stability. We show that these size distortions can become so large that a true null is rejected with certainty. The first main aim of this paper is to quantify these size distortions for structural break tests that are applied conditional on large deviations being observed in the data. Of course, such tests are exclusively constructed to hold size unconditionally and, hence, suffer from size distortions if applied otherwise. Hence, any change point test, if it is to be valid, needs to hold size conditional on having looked at the data. However, one problem with the recommendation to look for ‘any apparent sharp changes in behavior’ is that the decision to apply the structural break test has been informed by the data. To avoid such misleading test results, applying a formal structural break test is typically recommended as a pre-step to the actual statistical analysis of the data. For instance, Xu ( 2015) shows that if a structural break in the error variances is ignored, standard tests for the the constancy of regression coefficients suffer from size distortions-even asymptotically. 2013 Demetrescu and Hanck 2013 Harvey et al. If such a change is present in the data, yet is ignored in the subsequent analysis, the conclusions drawn from the data may be invalid (see, e.g., Baltagi et al. For instance, Brockwell and Davis ( 2016, p. 12) recommend time series plots to check whether there are ‘any apparent sharp changes in behavior’. The importance of plotting the data as a first step of a statistical analysis is stressed in numerous textbooks (e.g., Ruppert and Matteson 2015 Brockwell and Davis 2016).
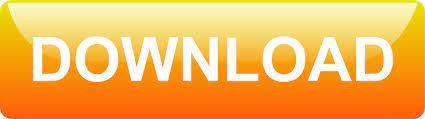